First came the computers. The idea of making machines as intelligent as humans followed soon after. The Theseus system, built by Claude Shannon, was one of the first attempts to develop intelligence in machines by creating a remote-controlled mouse that could remember its course and find its way out of a maze. Although simple, this humble beginning set the stage for a decades-long pursuit by humans to make machines "like us." But first, a benchmark for what like us means would have to be set.
The Turing Test for Artificial Intelligence
Initially, the Turing test, by mathematician and computing pioneer Alan Turing, was the accepted protocol. It evaluated whether a computer could be "smart" enough to be indecipherable from a human. It is a game in which an interrogator poses questions to a computer and a human; the idea is for the interrogator to determine which is human based on their responses. The computer wins/passes the Turing test if it fools the integrator. No computer has yet passed the test, but some have come pretty close.
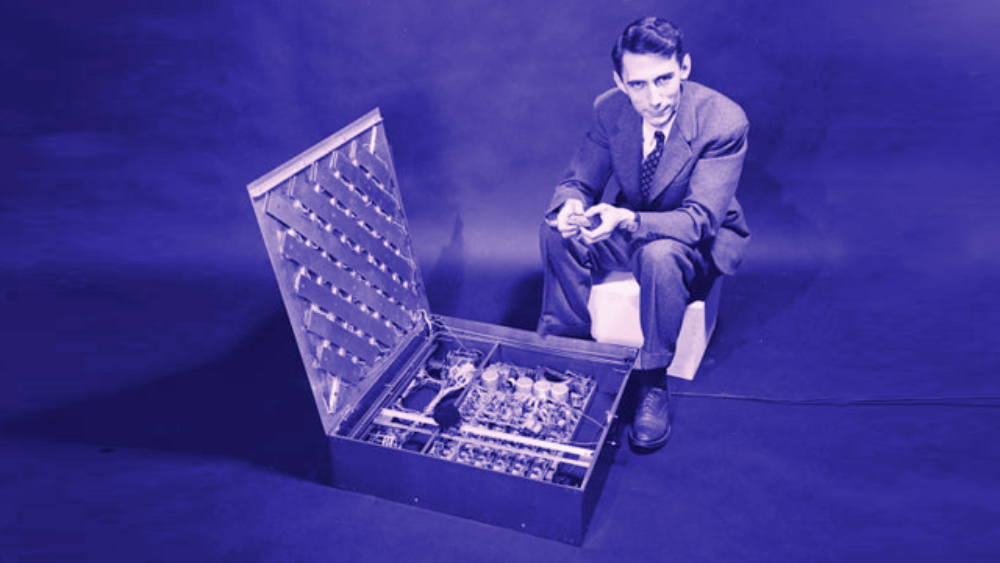
Intelligence Domains and What They Mean for AI
Beginning around the turn of the 21st century, more advanced tests were developed to test machines against human performance in five domains: handwriting recognition, speech recognition, image recognition, reading comprehension, and language understanding. A benchmark of zero was set as human-level performance. If you look at a graph of computer performance in these intelligence domains, you will see them rise steadily throughout the early 2000s. They converge to meet human capabilities from about 2015-2020. Since then, Ai systems have outperformed humans in all five domains.
It is crucial to keep in mind that performances like these were only on standardized tests. In real-world applications, the results are still mixed but steadily improving. However, the convergence of AI and Machine Learning brought us to where we are today with the explosion of generative AI.
How Machine Learning Relates to Generative AI
Generative AI —a branch of artificial intelligence— uses machines to create pictures, human-like conversations, designs, videos, and even computer code. It does this through machine learning, a computer science niche that has existed since about the same time as artificial intelligence. This discipline, which uses algorithms to "teach" computers how to learn from data without being programmed, took a little longer to progress. It wasn't until the 1980s that researchers developed algorithms called neural networks, which essentially mimic the structure and performance of a human brain. This makes them highly trainable.
However, it wasn't until computing power caught up to these discoveries in the 1990s that generative AI became more practical. First would come support vector machines (SVMs)which are supervised learning models that analyze data for classification and regression analysis. Next is deep learning, a type of layered neural network that allows for more complex data recognition. Deep learning has been instrumental in creating the advanced generative technology we see emerging today.
The Explosion of Generative AI Technology
In the last decade, generative AI product development has reached a dizzying pace. One of the most interesting (and essential) developments was Generative Adversarial Networks (GANs). Introduced by Ian Goodfellow and his colleagues in 2014, GANs are actually made up of two neural networks: a generator network and a discriminator network. They are trained in two separate competing ways. First, the generator network is trained to generate data, while the discriminator network is trained to discriminate between real and fake data. This so-called competition drives results from the AI generators that are increasingly indistinguishable from real, human-generated content.

The Future of Generative AI
Generative AI is remarkable and has done quite a bit to change the world we live in in the last few years. The future for generative AI is definitely integration into our daily lives. Things like Smart homes and self-driving are good examples of this and will no doubt be using generative AI technologies.
The Perils of Generative AI and How to Prevent Them
However, it is essential to remember generative AI presents scores of new risks and challenges. One of the most significant has to do with bias in AI systems. Since these models are fed vast amounts of information to "learn" from, the risk is that bias present in the data will be transferred to the AI databases, resulting in discriminatory outcomes. It is a garbage in, garbage out phenomenon. To avoid this, humans will need to find a way to supervise the data being used to train AI. This may look like globally accepted standards for transparency and accountability.
Deepfakes are another challenge regarding generative AI. There are already plenty of deep fakes out there that are reasonably convincing. This type of misinformation can have dire consequences. It can be used to manipulate public opinion or to generate widespread disinformation. It is clear from the bad actors already using generative AI for nefarious ends that international regulations need to be put in place fast.
However, through a human-centered approach and with the proper design thinking and regulatory framework, generative AI can avoid many of the pitfalls this type of technology invites and can be a way to improve the human experience through the use of intelligent machines.
Speck Design is a full service product design agency in the heart of San Jose. See some of our work below.